Evaluating Gesture Generation in a Large-Scale Open Challenge: The GENEA Challenge 2022
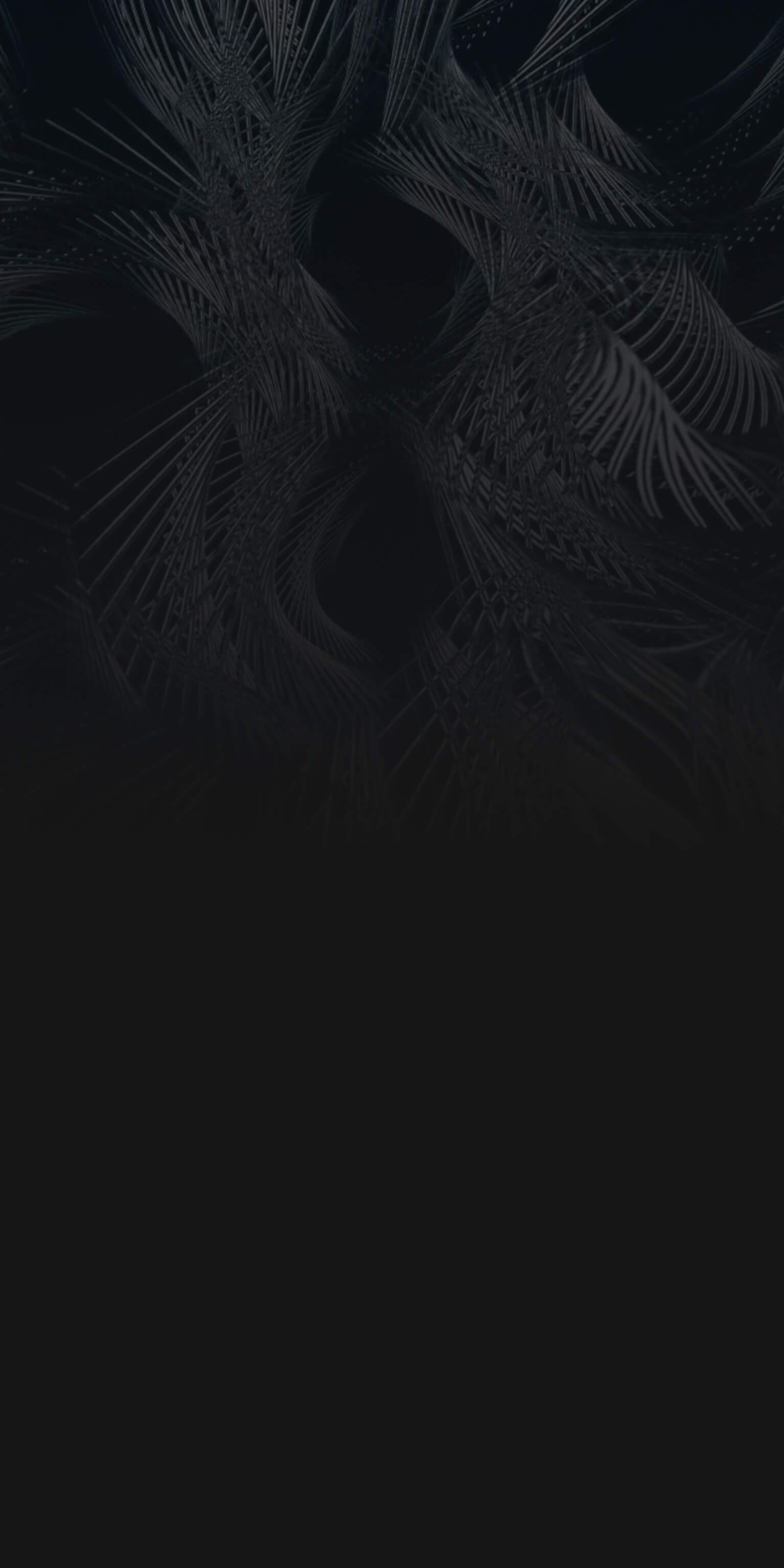
This paper was published in ACM Transactions on Graphics, April 2024.
Authors: Taras Kucherenko, Pieter Wolfert, Youngwoo Yoon, Carla Viegas, Teodor Nikolov, Mihail Tsakov, and Gustav Eje Henter
Evaluating Gesture Generation in a Large-Scale Open Challenge: The GENEA Challenge 2022
Download the paper (PDF 1.4 MB).
This paper reports on the second GENEA Challenge, a project to benchmark data-driven automatic co-speech gesture generation.
The participating teams in the challenge used a common speech and motion dataset to build gesture-generation systems. Motion generated by all these systems was rendered to video using a standardized visualization pipeline and evaluated in several large, crowdsourced user studies. Consequently, any differences in results are due only to differences between methods, enabling direct comparison between systems.
The dataset was based on 18 hours of full-body motion capture, including fingers, featuring different persons engaging in a dyadic conversation. Ten teams participated in the challenge across two tiers: full-body and upper-body gesticulation. For each tier, we evaluated both the human-likeness of the gesture motion and its appropriateness for the specific speech signal. Our evaluations decouple human-likeness from gesture appropriateness, which has been a difficult problem in the field.
The evaluation results show that some synthetic gesture conditions were rated as significantly more human-like than 3D human motion capture. To the best of our knowledge, this has not been demonstrated before. On the other hand, all synthetic motion is found to be vastly less appropriate for the speech than the original motion-capture recordings. We also find that conventional objective metrics do not correlate well with subjective human-likeness ratings in this large evaluation. The one exception is the Fréchet gesture distance (FGD), which achieves a Kendall tau rank correlation of around –0.5. Based on the challenge results, we formulate numerous recommendations for system building and evaluation.
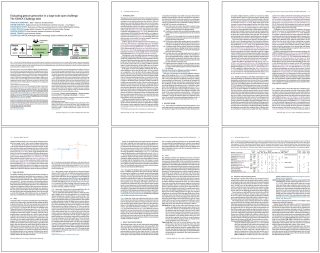
